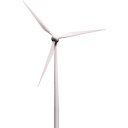
Forecasting Intermittent Wind and Solar Power
Business Challenge

Power producers and electric utilities must forecast production of electricity in order to meet their financial / physical production obligations. Wind and solar production is intermittent and with the growth of renewables the failure to perform can be quite costly due to imbalance costs/ fines and other penalties from contractual commitments to clients/ counterparties, as well as, producing grid unreliability and risks for electric customers.
Solution Considerations
Unlike other AI and machine learning projects the key to successfully forecast renewable power is the amount, quality and sources of data that are relevant to the problem at hand. Weather forecasts are a traditional source of data for this business challenge but weather is also notoriously hard to predict and has complex behaviour that makes it quite dynamic. As a result, various weather prediction models are commonly used to identify situations where forecasts can be enhanced. However, new data is becoming available that can be leveraged by the power industry to improve the accuracy of power production from renewable assets.
Differentiator
Airosa Limited is comprised of energy professionals that understand the power industry and is committed to bringing unique perspectives to the problem of forecasting renewable power. We understand weather is the starting point but is committed on improving forecast accuracy by adopting the latest data sets and modelling techniques to meet the needs of industry participants.
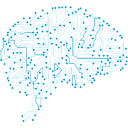
Forecasting Energy Prices
Business Challenge
Producers, utilities, consumers and traders need price forecasts for various reasons but primarily to enhance their financial performance, either to manage risk or simply to profit. Unlike forward curves, which give a picture of prices today for delivery in the future based on currently known information including a consensus view of unknowns, forecasts include a vast array of assumptions including risk appetite and other variables in order to anticipate where prices will out-turn in the future; as opposed to what they are worth today. Energy prices can be many thing to many people, whether that is the variation of price within day, day-ahead or the monthly and annual prices, for a specific demand/load shape, or simply a specified period of baseload energy. Long term forecasted prices may not move significantly day-day whereas nearby prices are more volatile; responding to new information and data that impacts sentiment as well as the underlying fundamentals.
Solution Considerations
Changing information with lots of data impacts, present good opportunities to be leveraged by AI and machine learning to improve the accuracy of energy price forecasts. When that information is sometimes more relevant than at other times, codifying the algorithms to learn when such data can be significant, allowing current market knowledge to modify forecasts of nearby energy prices.
Differentiator
Airosa Limited is comprised of energy professionals and a network of AI specialists that can translate the energy market information, when enhanced with AI and machine learning techniques, to meet the bespoke requirements of trading companies and those with a real need to understand nearby energy market movements.
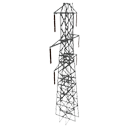
Forecasting Energy Consumption
Business Challenge
In European Union countries, energy consumption in buildings represents about 40% of the total energy consumption. In the USA, more than 44% of domestic energy consumption corresponds to heating, ventilating and air conditioning (HVAC) systems in buildings. Studies have shown that by following the current energy consumption pattern, the world energy consumption may increase more than 50% before 2030. Energy consumption management is a very significant problem not only to tackle the losses resulting from increasing consumption patterns but also to improve the performance of building energy systems.
Solution Considerations
The prediction of energy usage in buildings and modelling the behaviour of the corresponding energy system, are complicated tasks due to influential factors such as weather variables, building construction, thermal properties of the physical materials and occupants’ activities. Since the energy consumption process has a nonlinear behaviour, Deep Learning algorithms such as Artificial Neural Networks (ANN’s) are an Ideal fit for this problem due to their ability to handle a variety of data types. They are also quicker and easier to develop than engineering and statistical methods, while being accurate estimators. With sufficient historical data we are able to provide more accurate models and predictors.
Differentiator
Airosa Ltd, Having a team of energy professionals who understand the engineering challenges within a business and financial impacts of markets as well as environmental concerns along with a network of AI and machine Learning specialists creates a perfect storm to help businesses understand historic trends, current positions and a better visualisation of the future.
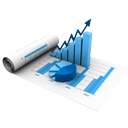
Enhancing Energy Trade Surveillance Systems detective measures via AI
Business Challenge
There are several new regulations (such as EMIR, MAD, REMIT, MAD2 and MAR) where some regulations are tackling market abuse with trade reporting requirements. Market abuse broadly consists of insider trading and market manipulation. In Energy, regulations now cover both physical, financial contracts, and transportation of commodities. Regulations compliance and enforcement are signalling for the adoption/ need for robust trade surveillance programs.
Solution Considerations
Leveraging AI and machine learning can improve the early identification of risky situations such as rogue traders, can credibly improve reputational image to regulators, counterparties and clients, can serve as strong internal deterrent to staff from non-compliance activities, and can increase visibility and company insights from internally generated information. Key to developing a comprehensive approach is reducing manual processes through to automation of detection via AI, and test for changing scenario’s which identify unusual trading patterns or flag potential problems with pre-trade, post- trade, and market pricing.
Differentiator
The key to a successful trade surveillance system is not having too many false-positives as well as not creating false negatives that dismiss possible infractions. Using a one standard policy will not be effective by flagging one desk too much and another not enough creating a lack of confidence in the program. The proper use of AI and machine learning is vital in the calibration process. Clearly a sensitivity analysis needs to be incorporated with some of the data. Our deep expertise in energy make us an ideal partner in an AI trade surveillance project.
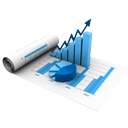
Trading Strategies
Business Challenge
The energy trading business around the world is constantly evolving with both regulation and deregulation policies as big drivers. The commodities industry has traditionally lagged the financial services and capital markets adoption of such things like appropriate governance, risk management practices and development of unique trades such as structured products. Leveraging technology, machine learning and artificial intelligence has also been slow to improve the operations, profits and efficiency of the commodities industry as a whole.
Solution Considerations
The central challenge to hedge funds, trading firms and asset management firms in the energy space in Europe is the raising costs due to inefficient operations and the increasing regulatory environment. This negative business effect is further compounded by low market prices, poor market liquidity and an unwillingness to risk reputational risk from poor performance on speculative trading.
Differentiator
Airosa believes the time is right to leverage machine learning and artificial intelligence to solve some the various challenges endured by trading houses, especially in Europe where free markets exist (compared to Asia where deregulation is ongoing). A push to trading on public exchanges which promote price discovery, the enhancement of market coupling in sectors like Gas & Power, and the increased pressure to generate profits above and beyond traditional asset optimization is amplifying the need to rely on unique trading strategies to uncover arbitrage opportunities, validate long or short position taking by identifying dependable technical analysis metrics/ tools with increased participation of Switzerland based hedge funds and identifying key changes to price and market trends via fundamental factors.
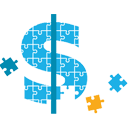
Energy Trading Capital Requirements
Business Challenge
The energy industry has had some fundamental changes in the way utilities, speculators, and asset management firms trade gas and power. Based on the push by various regulations and the need for enhanced transparency, reduced market manipulation incidents and reduced counterparty exposure and default risk participants have had an increased need for providing collateral for open positions. The migration of trading business to public exchanges and to Central Counterparty Clearing houses (CCP) for OTC based instruments has increased the need to manage cash and other collateral to meet initial and variation margin requirements.
Solution Considerations
Although exchanges and CCP’s can help money move more efficiently among traders by reducing the number of transactions being settled, which helps operations run more smoothly and reduces the value of obligations being settled, firms must rely on key data and tools to anticipate current and future capital requirements.
Differentiator
The key why Airosa is an ideal partner for modelling these type requirements is the enormity of the problem and its complexity by dealing with linear and non-linear instruments like options in anticipating possible mark to market changes and subsequent margin calls, understanding price volatility, behaviours and forecasting them accurately, properly modelling various exchanges and CCP margin logic and calculating available cash from operations to identify shortfalls and rely on possible bank relationships.